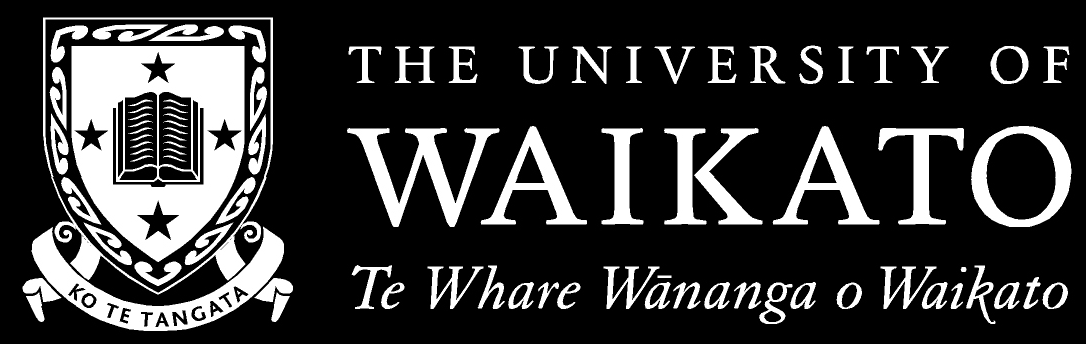
BUSAN302-23B (HAM)
Data-Informed Decision Making
15 Points
Staff
Convenor(s)
Pei-Chi Kelly Hsiao
6767
MSB.3.20
pei-chikelly.hsiao@waikato.ac.nz
|
|
Administrator(s)
You can contact staff by:
- Calling +64 7 838 4466 select option 1, then enter the extension.
-
Extensions starting with 4, 5, 9 or 3 can also be direct dialled:
- For extensions starting with 4: dial +64 7 838 extension.
- For extensions starting with 5: dial +64 7 858 extension.
- For extensions starting with 9: dial +64 7 837 extension.
- For extensions starting with 3: dial +64 7 2620 + the last 3 digits of the extension e.g. 3123 = +64 7 262 0123.
What this paper is about
How this paper will be taught
BUSAN302-23B (HAM) is offered in FLEXI Synchronous mode, which means some flexible elements of this paper can be completed online. Please note that you are required to engage in labs and scheduled assignments at set times.
Lectures:
- Week 1: Class on Monday. Orientation lecture on Monday that introduces you to the course, answer any questions you may have, and demonstrate the technical tools we will use for data analysis.
- Week 2 to Week 13: Classes on Friday only. No lectures on Monday, these are substituted for pre-recorded videos that covers the base concepts - make sure to review these prior to the labs as we will be applying those concepts.
- Live lectures will be live streamed via Panopto, recorded, and made available to all students. It is the sole responsibility of any absentees to catch up with missed content.
- We will be using R and Power BI Desktop throughout the course. Students should install these free software on their personal computer. Alternatively, students can remote access computer labs to use these software.
Labs:
- Labs commence in Week 2 and all sessions are delivered on-campus face-to-face and live streamed via Zoom.
- The lab sessions are not recorded.
- Students are encouraged to attend the sessions on-campus as it involves group work.
Required Readings
There is no required textbook. Recommended readings will be indicated and available on the course Moodle page, these include:
- Wickham, H. & Grolemund, G. (2016). R for Data Science. Available at: https://r4ds.had.co.nz/
- Silge, J. & Robinson, D. (2017). Text Mining with R: A Tidy Approach. Available at: https://www.tidytextmining.com/
- Boehmke, B. & Greenwell, B. (2020). Hands-On Machine Learning with R. Available at: https://bradleyboehmke.github.io/HOML/
Learning Outcomes
Students who successfully complete the course should be able to:
Assessments
How you will be assessed
The internal assessment/exam ratio (as stated in the University Calendar) is 100:0. There is no final exam.